CROSS-COUNTY SKIING
INTRODUCTION AND MOTIVATION
Analysis of the technique with PC application (task T2A): field trials have been carried out, both in outdoor conditions (Candachú, Belagua and Baqueira-Beret).
SPECIFIC OBJECTIVES
Implementation of the technique recognition algorithm (100%).
Technique analysis using the PC application (100%).
The trials for technique recognition were conducted at SnoZone (Madrid), analyzing 45 runs by two different subjects and applying machine learning techniques for technique recognition, see Figure 80% of the runs were used for system training, and the remaining 20% were passed to the artificial intelligence (AI) system for classification, achieving an accuracy rate exceeding 98% (see Figure B). This substantial improvement in results is mainly attributed to the enhanced electronic resolution, reducing temporal drift.
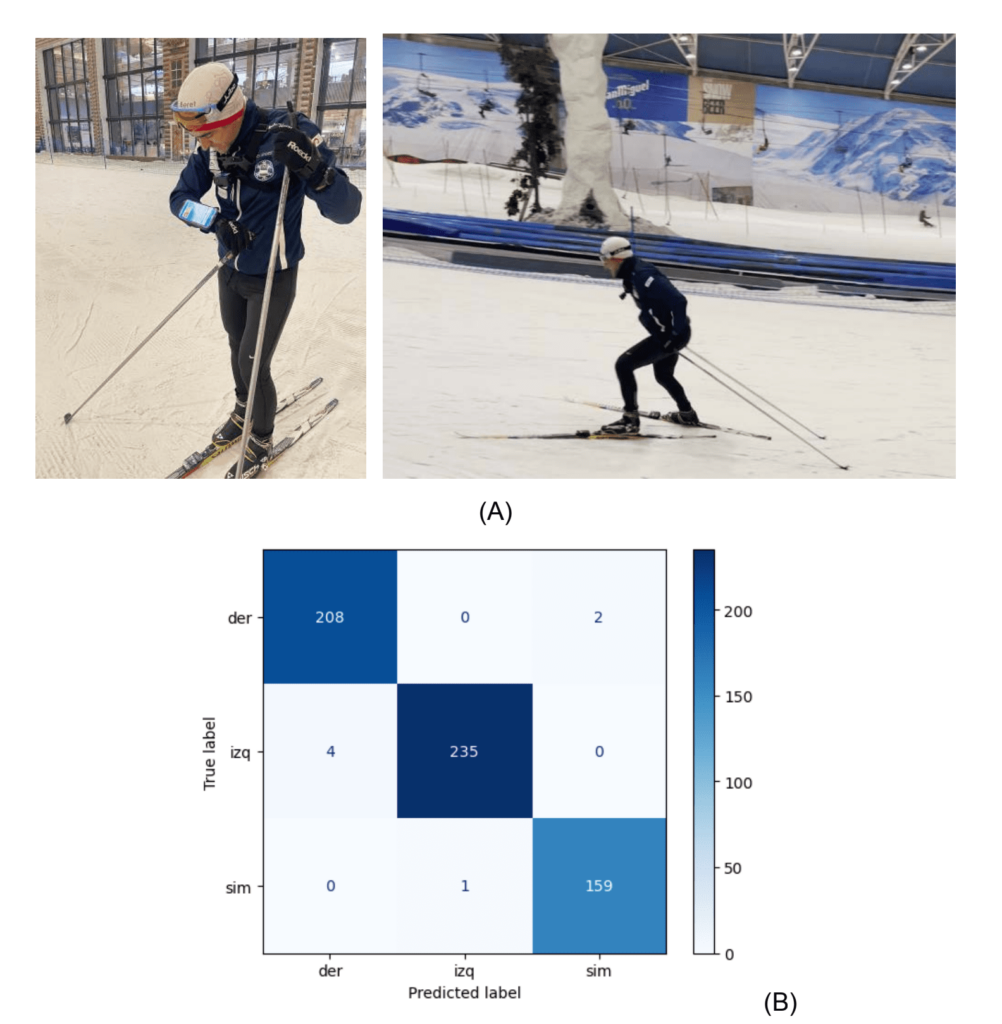
(A) Cross-country skiing trials conducted at SnoZone (Madrid). (B) Confusion matrix obtained after the application of artificial intelligence.
In the discipline of cross-country skiing, work has already begun with high-performance athletes, thus achieving the overall objective G4, as previously indicated:
G4. Generation of knowledge and transfer of research results to stakeholders (athletes, coaches, federations, and companies).
A prior study was conducted before the Spanish Championship in Tuixent (Lleida) with a high-performance athlete to analyze the symmetry of leg push in the classic style of roller skiing. For this purpose, a pair of sensor systems were placed on each roller ski, as shown in Figure. The results are presented in Figure, where a symmetrical leg push can be observed. A report has been sent to the regional federation coach and the athlete, both of whom have welcomed it very positively. This is the methodology that we aim to implement in various disciplines from this point forward.
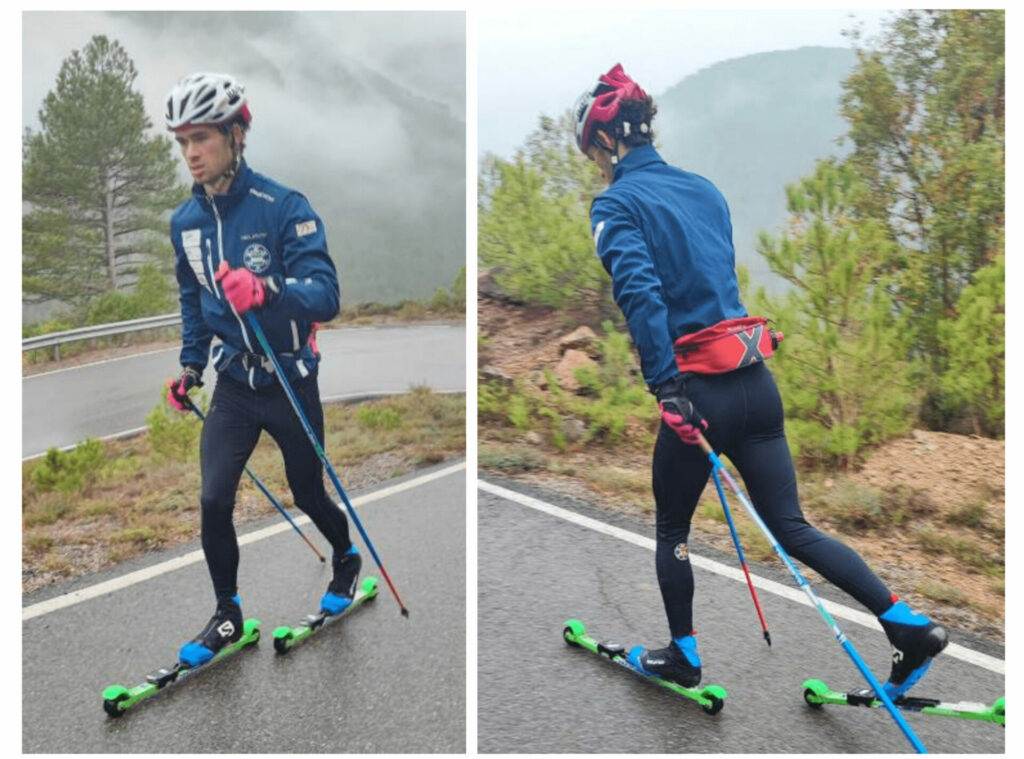
Symmetry Tests on Leg Push during Uphill Roller Skiing in Alternate Stride in Tuixent (Lleida).
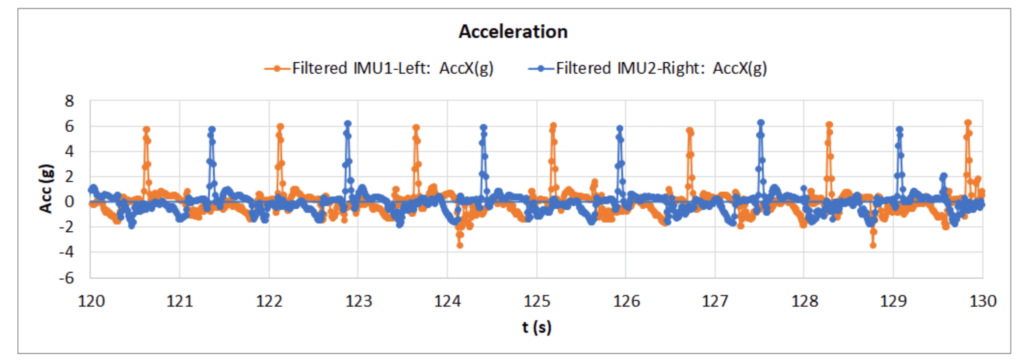
Acceleration in the direction of movement: In orange, the left roller, and in blue, the right roller.
DEVELOPED PROTOTYPE
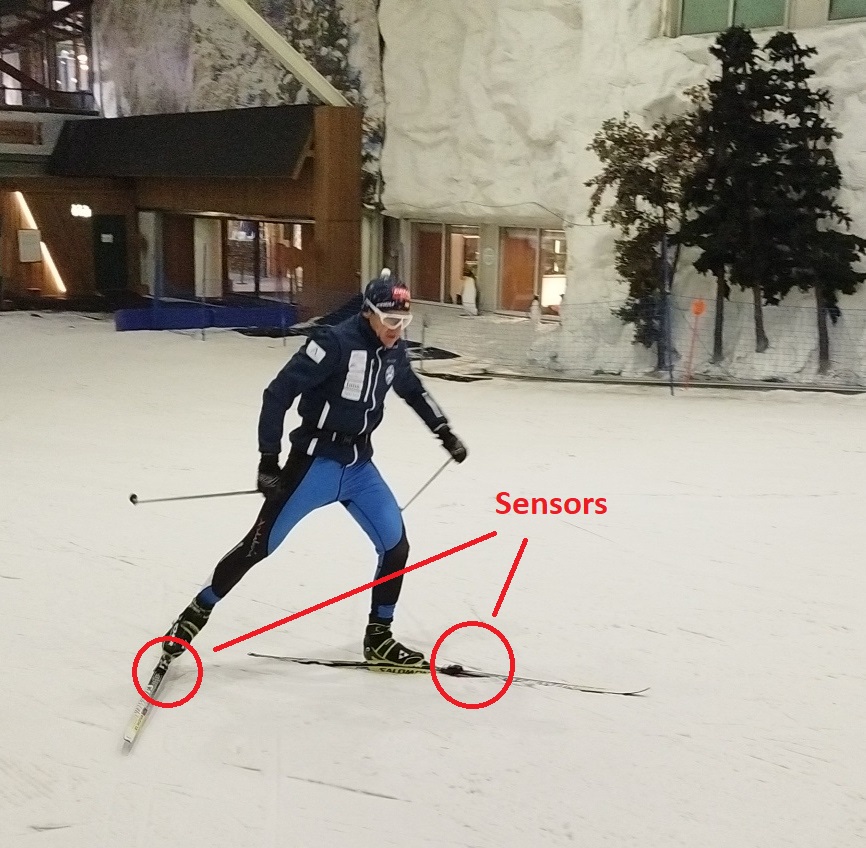
RESULTS AND CONCLUSIONS
The data provided by the inertial units placed on each of the skis and the videos made during the tests have been synchronized using the phyton application developed. Thanks to this analysis, it has been possible to create a database with the different climbing techniques in cross-country skiing and ski mountaineering for subsequent processing using artificial intelligence techniques. The following figure shows some of the results in the left asymmetric raise technique.
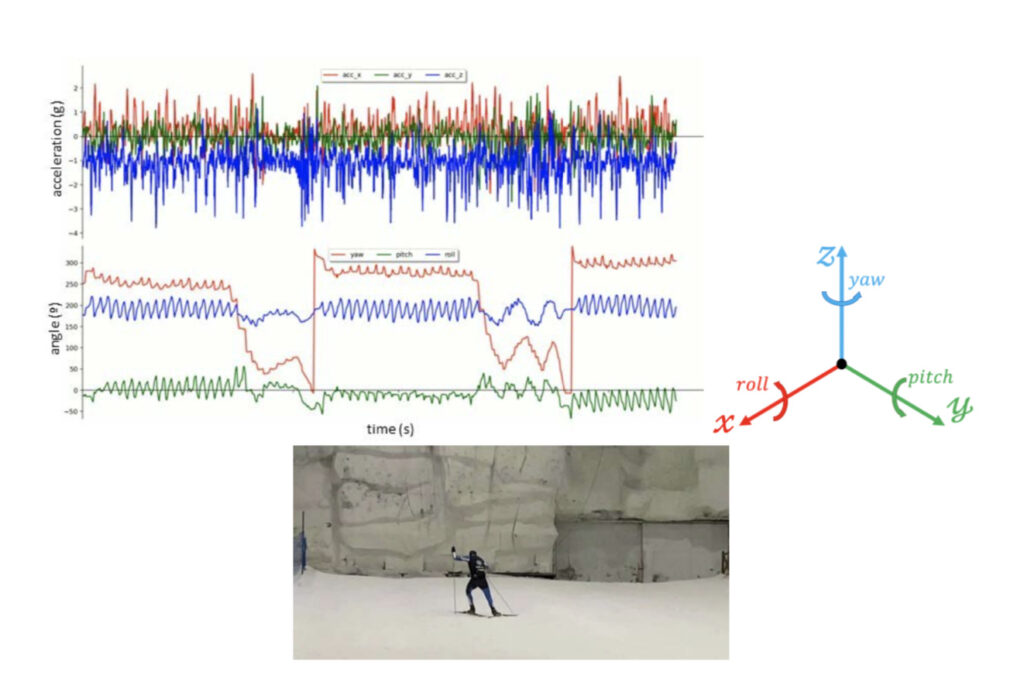
Example of video synchronization and inertial values for cross-country ski analysis for its analysis in left asymmetric uphill technique.
Implementation of the technique recognition algorithm (task T2A): a first technique classification engine based on machine learning has been developed that allows classifying the technique of climbing cross-country skiing in skating style, with a success rate of 94% in these first studies, which is very promising to continue with a larger number of trials and subjects. The previous Figure shows the results obtained using a concordance matrix.
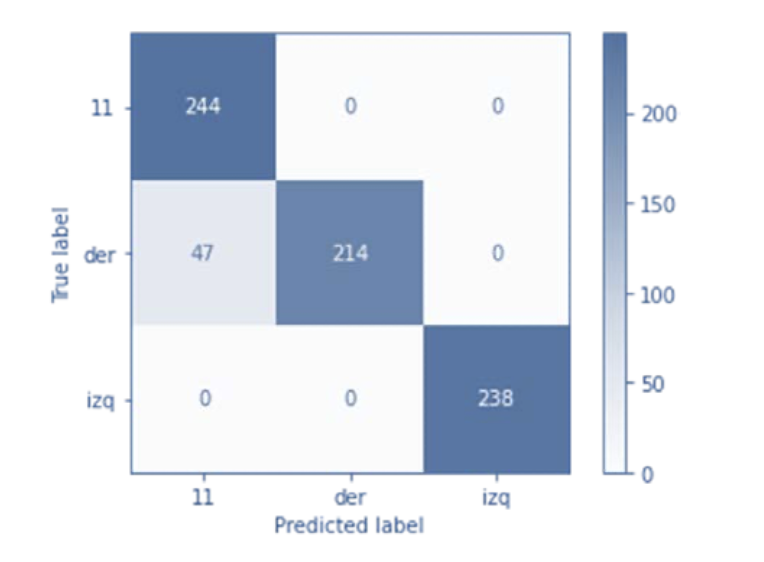
Concordance matrix between the real technique and the one predicted by a classification algorithm based on artificial intelligence.
In 2024, classic cross-country skiing reached new heights with validated data collection in Somport. Advanced AI algorithms now classify skiing techniques using integrated plantar pressure data. The database has expanded to include both indoor and outdoor inertial data, along with initial measurements for ski mountaineering.
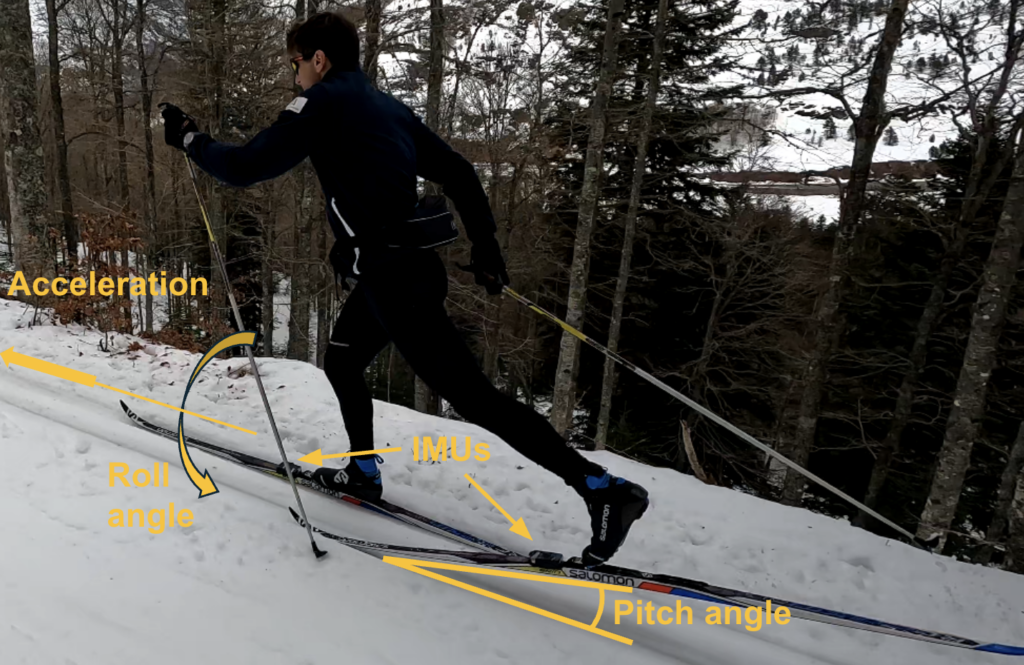
PEOPLE AND INSTITUTIONS INVOLVED
Isidoro Ruiz-García1
Ismael Navarro-Marchal2
Juan A. Moreno-Pérez1
P. Lorenzo Uhrich3
Alberto J. Palma1
Pablo J. Gómez-López2
Miguel A. Carvajal1
1 ECsens, Department of Electronics and Computer Technology, Sport and Health University Research Institute (iMUDS-UGR), Research Centre for Information and Communications Technologies (CITIC-UGR), University of Granada, 18071 Granada, Spain.
2 SkiingLab, iMUDS, Department of Physical and sport education, Sport and Health University Research Institute (iMUDS-UGR), University of Granada, 18007 Granada, Spain.
3Kustom Skis S.L., Calle Pérez Ayuso, 9, 28002 Madrid, Spain
This work was partly supported by Consejo Superior de Deportes through projects Project: “SensorSportLab III”, (Redes de Investigación en Ciencias del Deporte 2024) by Consejo Superior de Deportes (Ministerio de Cultura y Deporte), and European Regional Development Funds (ERDF).
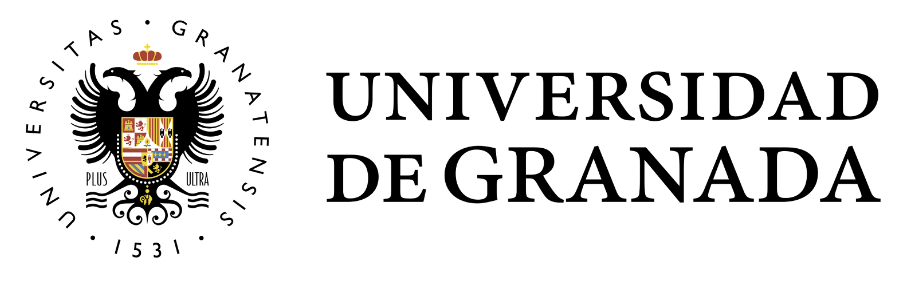
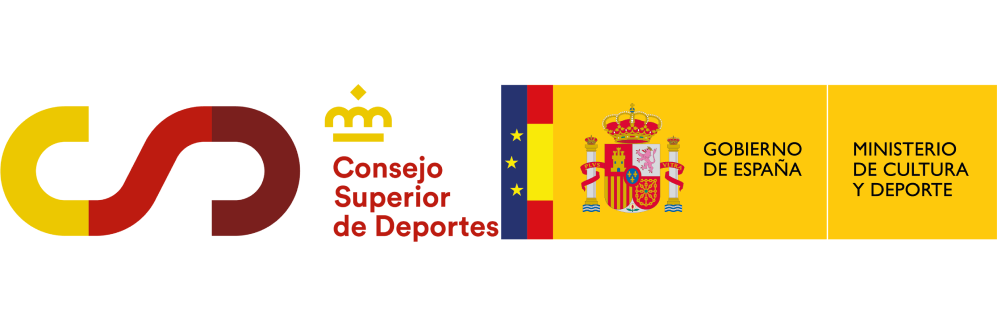
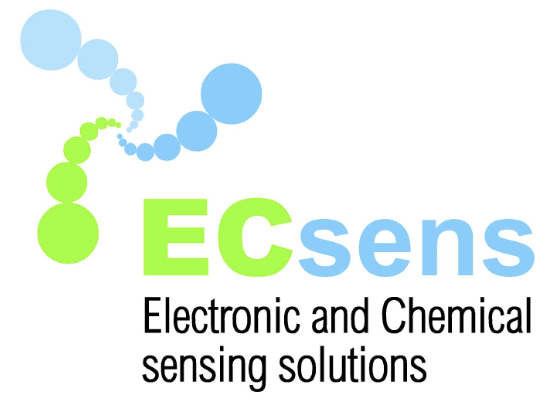